The environmental impact of LLMs: Here’s how OpenAI, DeepSeek, and Anthropic stack up
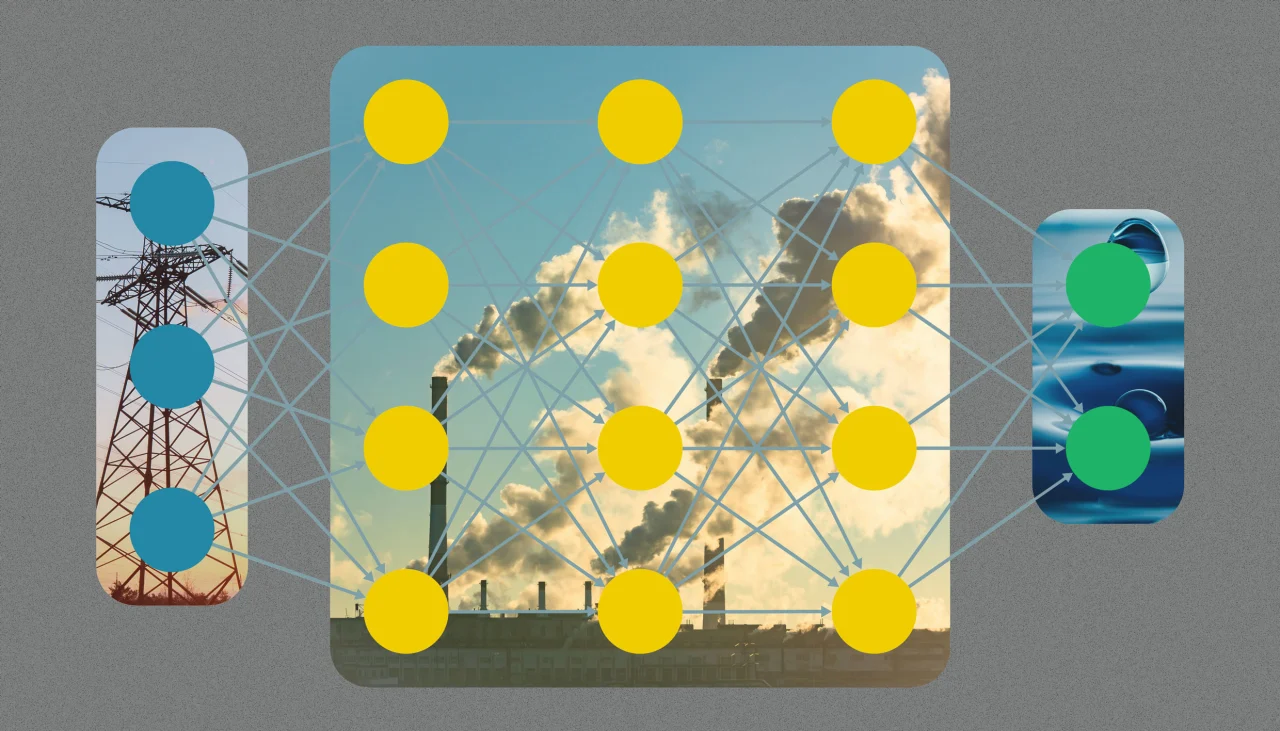
The companies behind AI models are keen to share granular data about their performance on benchmarks that demonstrate how well they operate. What they are less eager to disclose is information about their environmental impact.
In the absence of clear data, a number of estimates have circulated. However, a new study published in Cornell University’s preprint server arXiv offers a more accurate estimation of how AI usage affects the planet.
The research team—comprised of scientists from the University of Rhode Island, Providence College, and the University of Tunis in Tunisia—developed what they describe as the first infrastructure-aware benchmark for AI inference, or use. By combining public API latency data with clues about the GPUs operating behind the scenes and the makeup of regional power grids, they calculated the per-prompt environmental footprint for 30 mainstream AI models. Energy, water, and carbon data were then consolidated into an “eco-efficiency” score.
“We started to think about comparing these models in terms of environmental resources, water, energy, and carbon footprint,” says Abdeltawab Hendawi, assistant professor at the University of Rhode Island.
The findings are stark. OpenAI’s o3 model and DeepSeek’s main reasoning model use more than 33 watt-hours (Wh) for a long answer, which is more than 70 times the energy required by OpenAI’s smaller GPT-4.1 nano. Claude-3.7 Sonnet, developed by Anthropic, is the most eco-efficient, the researchers claim, noting that hardware plays a major role in the environmental impact of AI models: GPT-4o mini, which uses older A100 GPUs, draws more energy per query than the larger GPT-4o, which runs on the more advanced H100 chips.
The study also found that the longer the query, the greater the environmental toll. Even short queries consume a noticeable amount of energy. A single brief GPT-4o prompt uses about 0.43 Wh. At OpenAI’s estimated 700 million GPT-4o calls per day, the researchers say total energy use could reach between 392 and 463 gigawatt hours (GWh) annually—enough to power 35,000 American homes.
Individual users’ adoption of AI can quickly scale into significant environmental costs. “Using ChatGPT-4o annually consumes as much water as the drinking needs of 1.2 million people annually,” says Nidhal Jegham, a researcher at the University of Rhode Island and lead author of the study. “At a small scale, at a message or prompt scale, it looks small and insignificant, but once you scale it up, especially how much AI is expanding across indices, it’s really becoming a rising issue.”
What's Your Reaction?
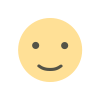
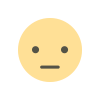
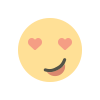
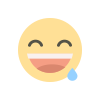
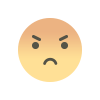
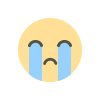
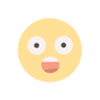