Trump’s war with universities could hurt AI progress in the U.S.
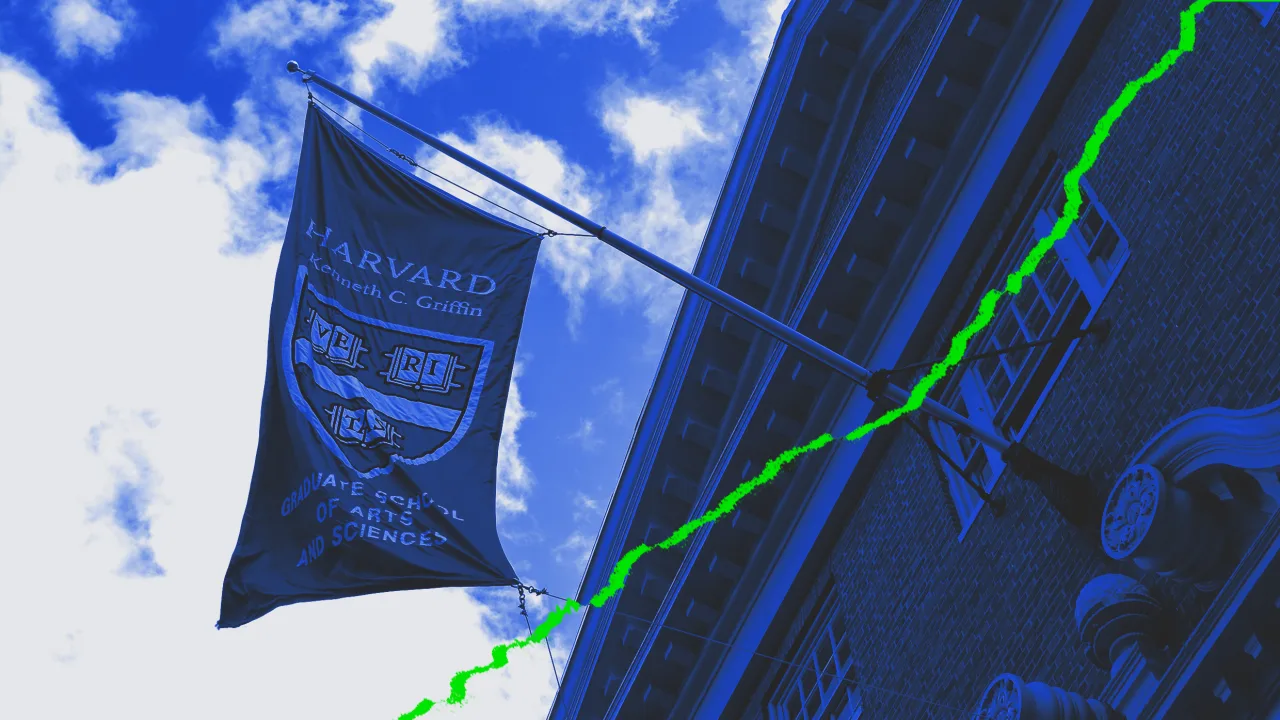
Welcome to AI Decoded, Fast Company’s weekly newsletter that breaks down the most important news in the world of AI. You can sign up to receive this newsletter every week here.
Trump harms AI progress by warring with universities
Donald Trump has done a lot to antagonize universities in his first 100 days. He cut off federal research funding to institutions like Princeton, Columbia, and Harvard, citing their alleged tolerance of antisemitism on campus. He also threatened the authority of college accreditation bodies that require schools to maintain diversity, equity, and inclusion programs. But these actions directly undermine the administration’s stated goals of strengthening the U.S. military and helping American tech companies maintain their narrow lead over China in AI research.
Since World War II, the U.S. government has maintained a deep and productive relationship with universities. Under the leadership of Vannevar Bush, director of the Office of Scientific Research and Development, the government channeled significant research funding into university labs. In return, it received breakthroughs like radar and nuclear technology. Over the decades, university researchers have continued to contribute critical innovations used in defense and intelligence, including GPS and the foundational technologies of the internet.
Today, the government increasingly relies on the commercial sector—including major contractors like Boeing and General Dynamics, and newer firms like Palantir and Anduril—for defense innovation. Yet universities remain essential. Much of the most advanced AI research still originates from academic computer science departments, many of which are powered by international students studying at institutions like MIT, Stanford, and Berkeley. These students often go on to found companies based on research initiated in academia. Whether they choose to build their businesses in the U.S. or return to their home countries depends, in part, on whether they feel welcome.
When international students see research funding threatened or videos of PhD students being arrested by ICE, staying in the U.S. becomes a less appealing option. In a recent conflict with Harvard, the Department of Homeland Security even demanded information on the university’s foreign students and threatened to revoke its eligibility to host them. In response, over 200 university and college presidents have condemned the administration’s actions and are exploring ways to resist further federal overreach.
Rather than discouraging international researchers and students, the U.S. should be sending a clear signal: that it remains a safe, supportive, and dynamic environment for AI talent to study, innovate, and launch the next generation of transformative companies.
The best AI agents may be powered by teams of AI models working together
During the first phase of the AI boom, labs achieved big intelligence gains by pretraining their models on ever-larger data sets and using more computing power. While AI companies are still improving on the art and science of pretraining, the intelligence gains are becoming increasingly expensive. A big part of the research community has shifted its focus to finding the best ways to train models to “think on their feet,” or to reason over the best routes to a responsive and accurate answer at “inference time” just after a user enters a question or problem. This research has already led to a new generation of “thinking” models such as OpenAI’s o3 models, Google’s Gemini 2.0, and Anthropic’s Claude 3.7 Sonnet. Researchers teach such models to think by giving them a multistep problem and offering them a reward (usually just a bit of code that means “good”) for finding their way to a satisfactory answer.
It’s certainly possible to build an inference system that makes numerous calls to a single large frontier AI model, collecting all the questions and answers in a “context window” as works toward an answer. But new research from Berkeley’s AI research lab shows this monolithic “one model to rule them all” approach is not always the best way of building an efficient and effective inference system. A “compound AI system” of multiple models, knowledge bases, and other tools working together can yield more relevant and accurate outputs at far lower costs. Importantly, such a “pipeline” of AI resources can be a powerful backend for AI agents capable of calling on tools and working autonomously, says Jared Quincy Davis of the AI cloud company Foundry. Foundry builds software that enables it to provide GPU compute at low cost for AI developers.
Davis has led an effort to create an open-source framework that lets AI practitioners build just the right pipeline, with just the right resources, for the application they have in mind. The framework, called Ember, was created with help from researchers at Databricks, Google, IBM, NVIDIA, Microsoft, Anyscale, Stanford, UC Berkeley, and MIT. Davis says it’s possible to build a compound system that can make calls to a number of today’s state-of-the-art AI models (via APIs) such as ones from Google, OpenAI, Anthropic, and others. Large frontier models often stand above other large models in certain skill areas (Anthropic’s Claude is especially good at writing and analyzing text), so it’s possible to build a pipeline that calls on models according to their unique strengths.
This is a very different way of looking at AI computing, compared to the narrative of just a couple years ago that said one model would be better than all others at practically everything. Now, numerous models compete for the state-of-the-art at various tasks, while other smaller models specialize in completing tasks at lower cost, and the overall cost of getting an answer from an AI model has gone way down over the past couple of years.
Congress actually passes a tech bill
Congress has failed to pass any broad-based regulation to protect user and data privacy on social networks. It has, however, managed to pass laws to prohibit specific and particularly dangerous social media content such as child sex trafficking, and now nonconsensual intimate images (or NCII).
NCII refers to the practice of posting sexual images or videos of real people online without their consent (often as an act of revenge or an attempt to extort), including explicit images generated using AI tools. The bill, called the Take It Down Act, which unanimously passed the Senate in February and the House on Monday, makes it a federal crime to post NCII and requires that online platforms remove the content within 48 hours of a complaint. Affected “public-facing” online platforms will have a year after the law passes to set up a system for receiving and acting on complaints. The president is expected to sign the bill into law.
Even though the bill’s intent earned widespread support, its legal reach disturbed some free expression advocates. The Electronic Frontier Foundation worries that the bill’s language is overbroad and that it could be used as a tool for censorship. These worries were compounded by the fact that the new law will be enforced by the Federal Trade Commission, which is now led by Trump loyalists.
More AI coverage from Fast Company:
- Duolingo doubles its language offerings with AI-built courses
- In his first 100 days, Trump’s tariffs are already threatening the AI boom
- Microsoft thinks AI colleagues are coming soon
- Marc Lore wants AI to feed you—and make you healthier
Want exclusive reporting and trend analysis on technology, business innovation, future of work, and design? Sign up for Fast Company Premium.
What's Your Reaction?
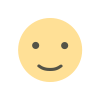
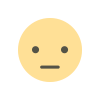
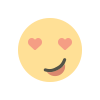
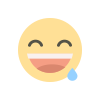
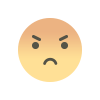
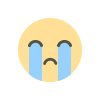
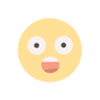